New machine based model developed may help fight sarcopenia by predicting the biological age of the muscle. Sarcopenia means loss of skeletal muscle.
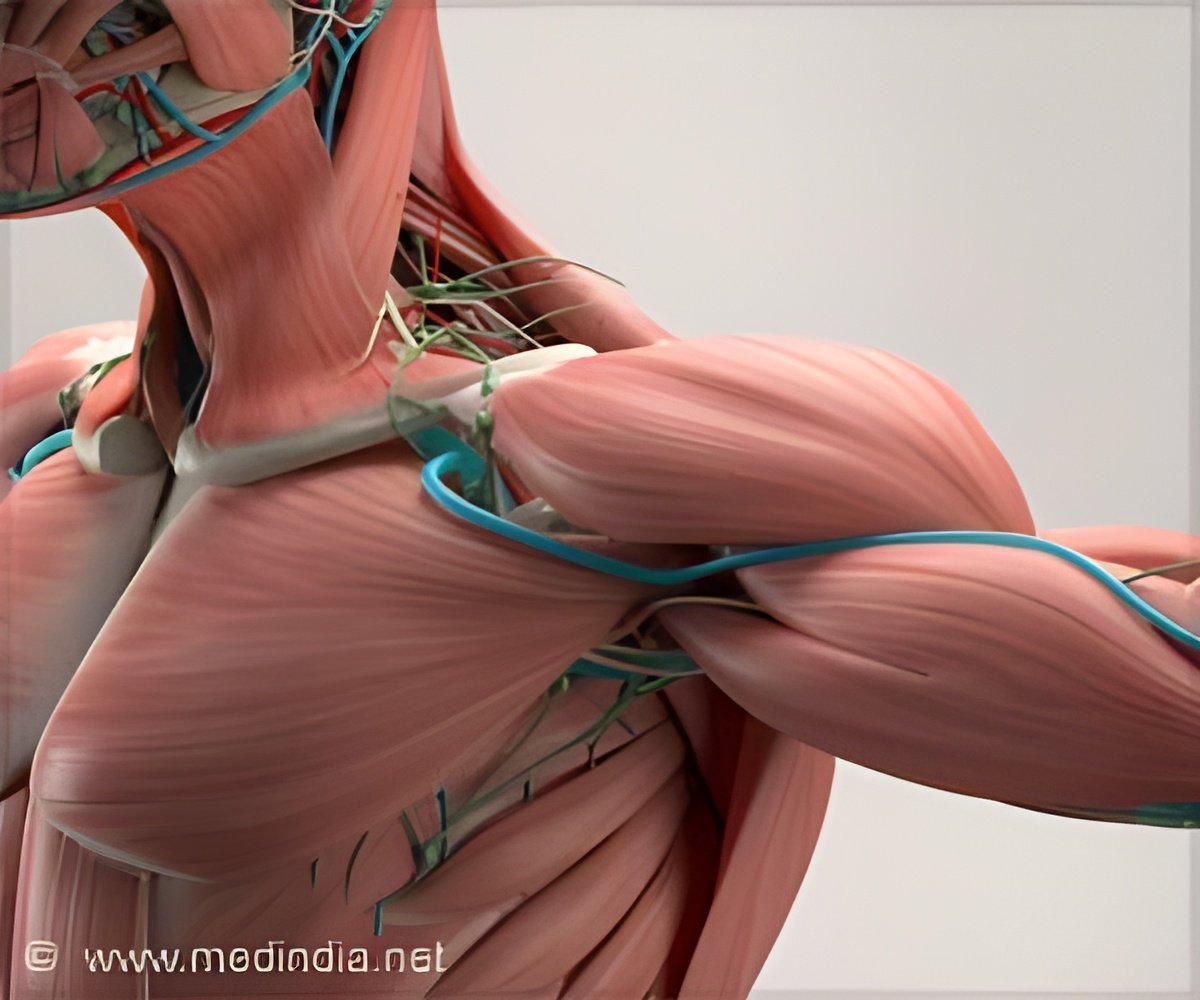
‘Understanding the molecular mechanisms of muscle aging can help discover potential treatment methods for sarcopenia (muscle wasting).’
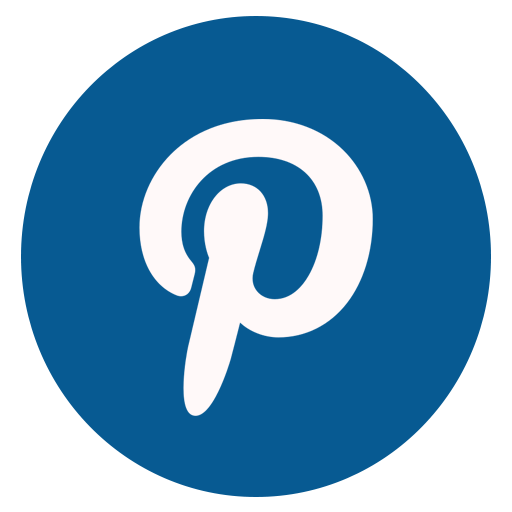
To address this challenge researchers from Insilico Medicine developed a novel deep-learning based model. "We are working on multiple biomarkers using deep learning and including blood biochemistry, transcriptomics, and even imaging data to be able to track the effectiveness of the various interventions we are developing. We believe that the most effective anti-aging therapy should be tissue-specific, so we focused on the development of tissue-specific biomarkers of aging. This work is an example of a marker of skeletal muscle tissue. Internally, we work with six tissues, including the liver, skin, and lungs." said Polina Mamoshina, senior deep learning scientist at Insilico Medicine.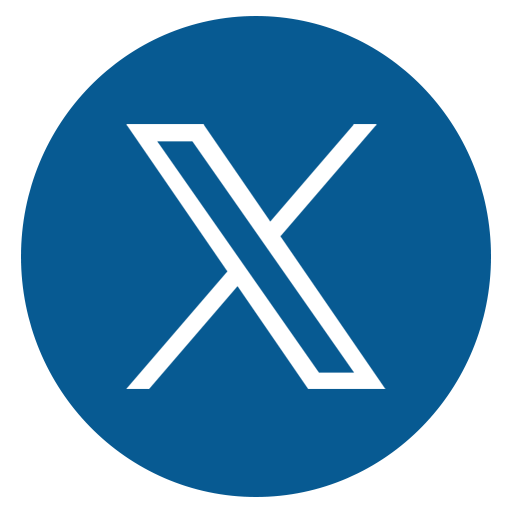
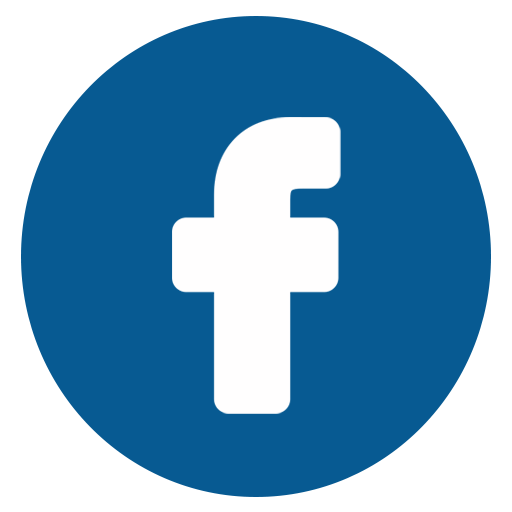
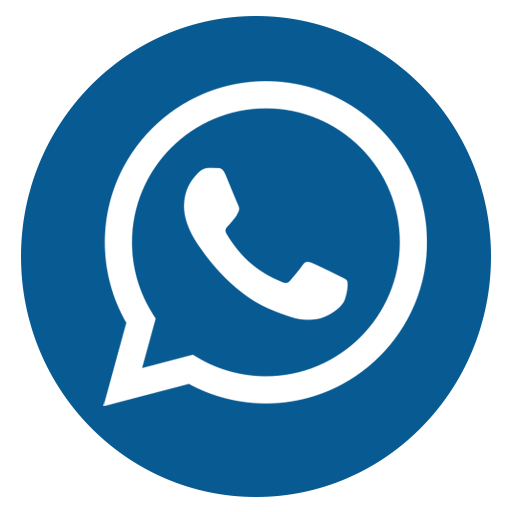
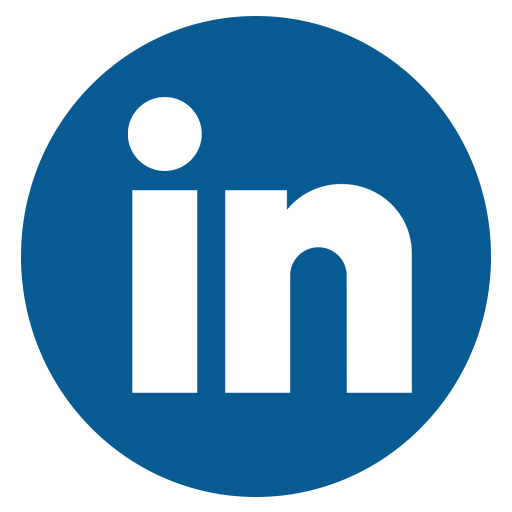
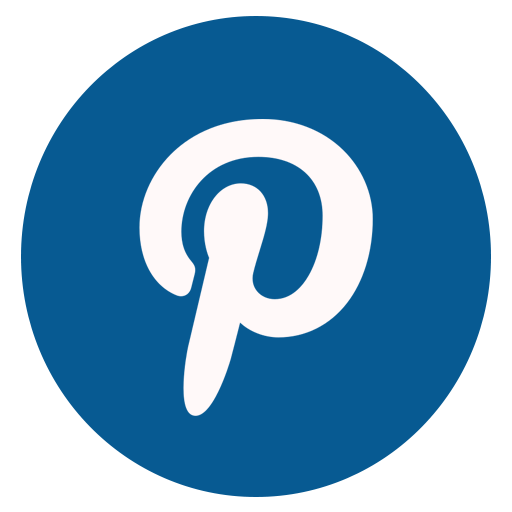
In this study, the scientists applied a state of the art signaling pathway analysis algorithm, iPANDA, to compare transcriptomic signatures of 'old' and 'young' tissues and utilized several machine learning methods to predict the age of samples based on their transcriptomic signatures. Ultimately, the trained age predictors were used to identify tissue-specific aging clocks.
This combined data-driven approach demonstrates that age prediction models can become a powerful tool for identifying prospective targets for geroprotectors.
"Sarcopenia is one of the lowest-hanging fruits and major commercial opportunities out of all of the diseases of aging we are focusing on. This paper presents one of the many way machine learning can be used to identify biological targets in muscle wasting. While this approach does not establish causality or evaluate the most important targets for a specific age group, it may be appealing to the pharmaceutical companies looking at developing broad-spectrum interventions for sarcopenia. I am very happy to see that Polina's work done in 2015 finally saw the light", said Alex Zhavoronkov, PhD, founder, and CEO of Insilico Medicine, Inc.
Advertisement